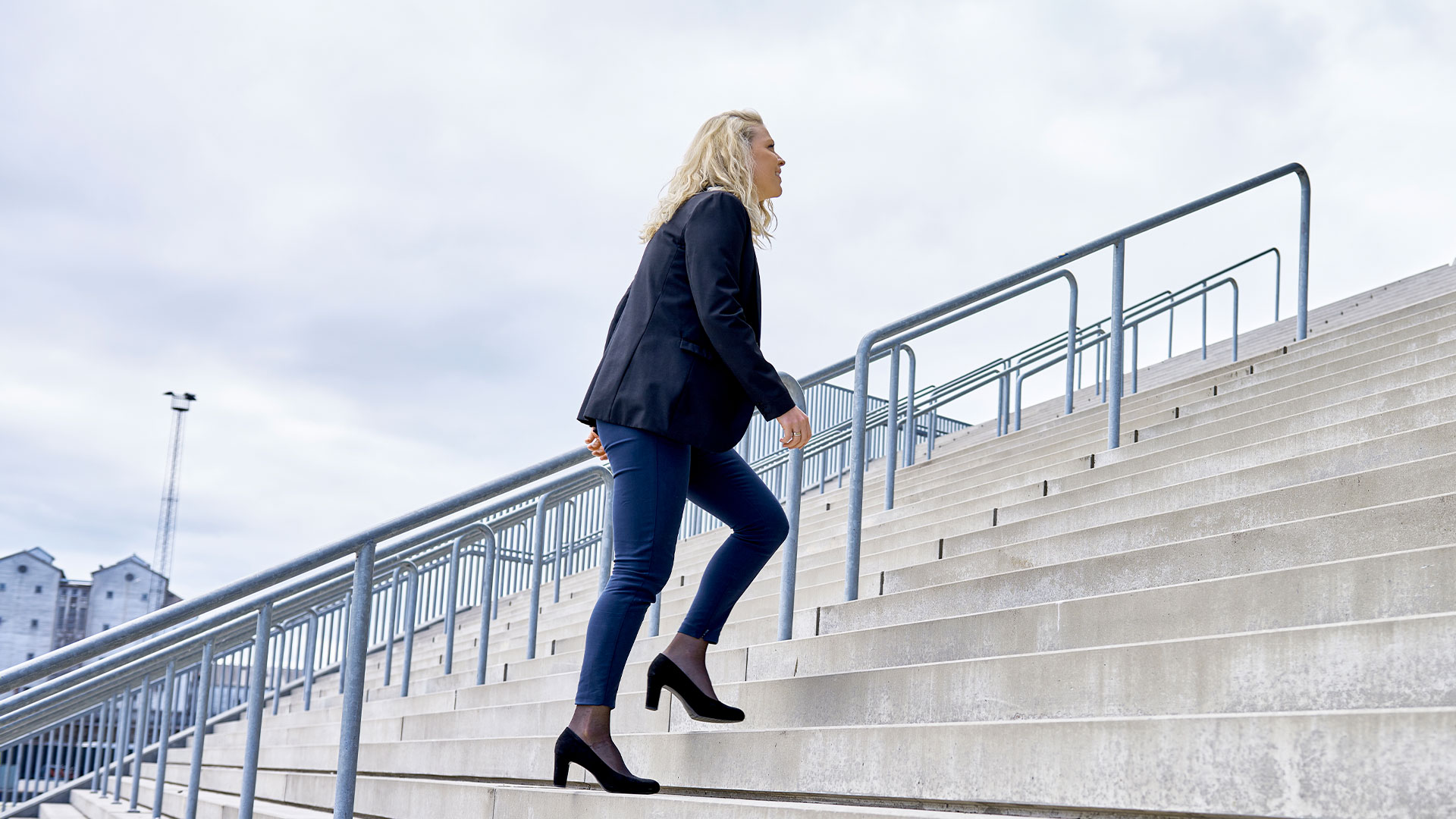
Sure, your customers love you and your company. Until that one day, when they don’t love you anymore – and they didn’t even leave a farewell letter before falling into the arms of your competitor. Perhaps you were busy acquiring new customers and generating new sales?
If you trust that your customers are loyal enough to call you and share thought of potentially leaving you, this is your exit from this blogpost. If you are looking for a heads up on customers at risk of churning, well in advance of the event taking place, please continue reading.
Where do you put your money?
Companies are often willing to invest in marketing, campaigns, leads and starting offers. However, some tend to underestimate what comes next. What comes next are all the possibilities to improve the entire customer journey and ensure continuously satisfied customers. Unfortunately, the time and investments going into these possibilities are peanuts compared to the acquisition phase. This could turn out to be a big mistake. Just think about all your hard work and huge investments going into getting the customer in the first place.
So how do you start focusing more on retaining your customers and where should you place your investments? My recommendation is to harvest all the delicious fruits produced by data and machine learning in this area. The most recognized, understandable and usable method to improving customer retention is churn prediction. Building a churn model provides you with insight into your business, helps you prevent customers from churning and generally improves customer satisfaction.
I do recognize that many of you are already looking into this, however, I’m going to challenge your perspective. It's not that you are choosing a wrong algorithm, writing unsustainable code or using the math in a wrong way. No, it’s the way you are thinking about the problem from a business perspective. And why do I know this, you may ask? Well, that’s because I have made all the mistakes myself in the past.
When a project is implemented, we use data to make predictions so we can act and prevent the event from happening. But when the project is scoped and defined, the process is reversed. At first, you should map down the actions as these need to be there before doing anything at all. Then you define your prediction target to justify the actions. In the end, we look at the data available.
The below is not rocket science but things you need to have a clear perspective on before embarking your churn adventure.
1. Actions are all the stuff you can do to prevent customers from churning. Somebody in your organization should be able to act on the model predictions and the task here is to map these actions. To do this, you must address and describe the following questions:
Who do we want to prevent from churning?
Machine learning tries to detect patterns by explaining a future churn. These patterns should be detected within groups of similar behavior to enable the best possible performance. We recommend narrowing down the customers of interest by answering:
- Are we trying to prevent private or business customers from churning?
- Can we classify our customers into smaller groups with similar behavior? This could be a split by country as an example.
- Do we need the customers to have a specific product mix?
Who is going to be the end-user of the prediction model?
After defining the customers of interest, it is easier to define the end-user. Let’s say the customers have been defined as private customers in Denmark and depending on your organization, the end-user could be Danish salespeople. From this point, we need to tailor a solution to the end-user:
- What is the preferred format to deliver the results? In this BI-saturated world, we no longer bother with dashboards. Even within the organization, we want a notification and a recommendation for what to do.
- How fast can the end-user act to prevent a churn? This question should be answered to establish a reasonable timeframe for the predictions. Do we need to predict the event one week in advance, one month in advance, two months in advance, etc.
- Should the end-user make use of the reasons behind the churn predictions? If the end-user can take actions upon specific explanatory factors, we recommend including the primary churn factors in the solution.
What actions can the end-user take to prevent a future churn?
This is about defining a clear strategy and making guidelines for the end-user. When the end-user receives the model predictions, what can then be done to keep the customer from churning or reactivate the customer:
- Should we contact the customer personally, digitally or a mix of both?
- Do we need to improve our time-to-delivery?
- Can we offer a certain amount of discount?
- Do we need a differentiated strategy towards the customers? Some customers can be preferred over others and should perhaps be offered a better discount, extra service, etc.
- Should we try to change the customer’ product mix by offering new products?
2. Predictions are probably the most overlooked and undervalued aspect when building a churn model. Unfortunately, the way machine learning is taught at universities and the way some people work on data science platforms such as Kaggle could leave one with the impression that a target is always served on a silver plate. Anyone working with machine learning in the real world knows this is not true. Defining a prediction target is in fact a tough and complicated task and many hours often fly by answering the below:
When is your customer about to leave you?
You need to find the point in time, where the customer takes a conscious decision to take the business elsewhere. This conscious decision is what we want to prevent from happening in the future.
I advise you to have your most skilled domain experts define the target. If the prediction target is chosen too late, we can’t prevent the event from happening. If it is too early, there will be too much noise around the target for the model to learn any significant patterns. You need to take this seriously!
3. Data can cause you a lot of pain, but if used in the right way, it can provide you with insight into patterns you never thought of. Let’s take a deep dive into the data lake by answering the following:
What data is relevant when detecting churn patterns?
Since we are taking a data approach to solve the problem, we are also limited by the data available. We will map down the entire process from a customer’s starting date and going forward. As a rule of thumb, we are always trying to include as much as possible. The most common areas and questions are stated below:
- Time. How far back in time do we have data? Can we tell when a customer became a customer for the first time? Do we have timestamps for all observations?
- Customer data. What kind of profile data do we have on our customer? This could be place of residence, age, gender etc.
- Historical transaction behavior. When and how many transactions/products?
- Contact points. When and how many times have we been in contact with the customer? This could be a sales phone call, check up call, training session, etc.
- Correspondence/papers. Do we have text resumes of prior conversations? Can we access the text in a complain?
What is your next move?
Hopefully, you’ve been inspired to expand your perspective and existing sales toolbox. If you are looking to get serious about machine learning, I recommend that you consider the following:
- Are you and your colleagues willing to put time and effort into a machine learning project?
- Do you have the internal resources to handle a machine learning project?
- Are you ready to get started?
Let us help you get started!
Now, we have gone through some of the main areas and considerations you need to cover before building a churn model. If you want to know more and get a better idea of how to succeed, we will take you through each of the steps described.
Please reach out to info@inspari.dk or +45 70 24 56 55 if you have any questions. We are looking forward to hearing from you.
Interested in a case study?
Read the case about the shipping company, Ziton, using predictive analytics to predict the future of off shore wind turbines in the North Sea.
Ziton case
Relaterede Posts
Don't drown the data - show it!
Far too often, we seem overly worried that our audience will not find our data interesting and...
Do you know C.R.A.P. when you see it?
If you popped by this blog, chances are that you work with BI or data analysis or just find...
QlikView - Loading images into memory
Images in a QlikView application can be power full in many ways. Most of the time I see solutions...